A vision transformer for decoding surgeon activity from surgical videos
Surgeon activity impacts patient outcomes
Over 300 million surgical procedures are performed worldwide by surgeons. Such surgeons’ actions during surgery, or intraoperative activity, can have a substantial impact on the outcome of a patient after surgery. Improving patient outcomes can therefore be achieved, at least in part, by modulating the behaviour of surgeons. Whereas optimal behaviour can be reinforced, subpar performance can be identified for continual improvement.
Details of surgeon activity remain elusive
Before we can modulate surgeon behaviour, we first need to quantify the core elements of intra-operative surgeon activity. This includes identifying what and how activity is performed by a surgeon during surgery. To understand the core elements of surgery, consider a robotic surgery known as a robot-assisted radical prostatectomy (RARP), in which a cancerous prostate gland is removed from a patient's body. This procedure consists of a sequence of steps over time, such as dissection (read: cutting tissue) and suturing (read: joining tissue), reflecting the what of surgery. Each step can be executed through a sequence of manoeuvres, or gestures, at varying skill levels, reflecting the how of surgery. Up until now, the details of such activity have remained elusive.
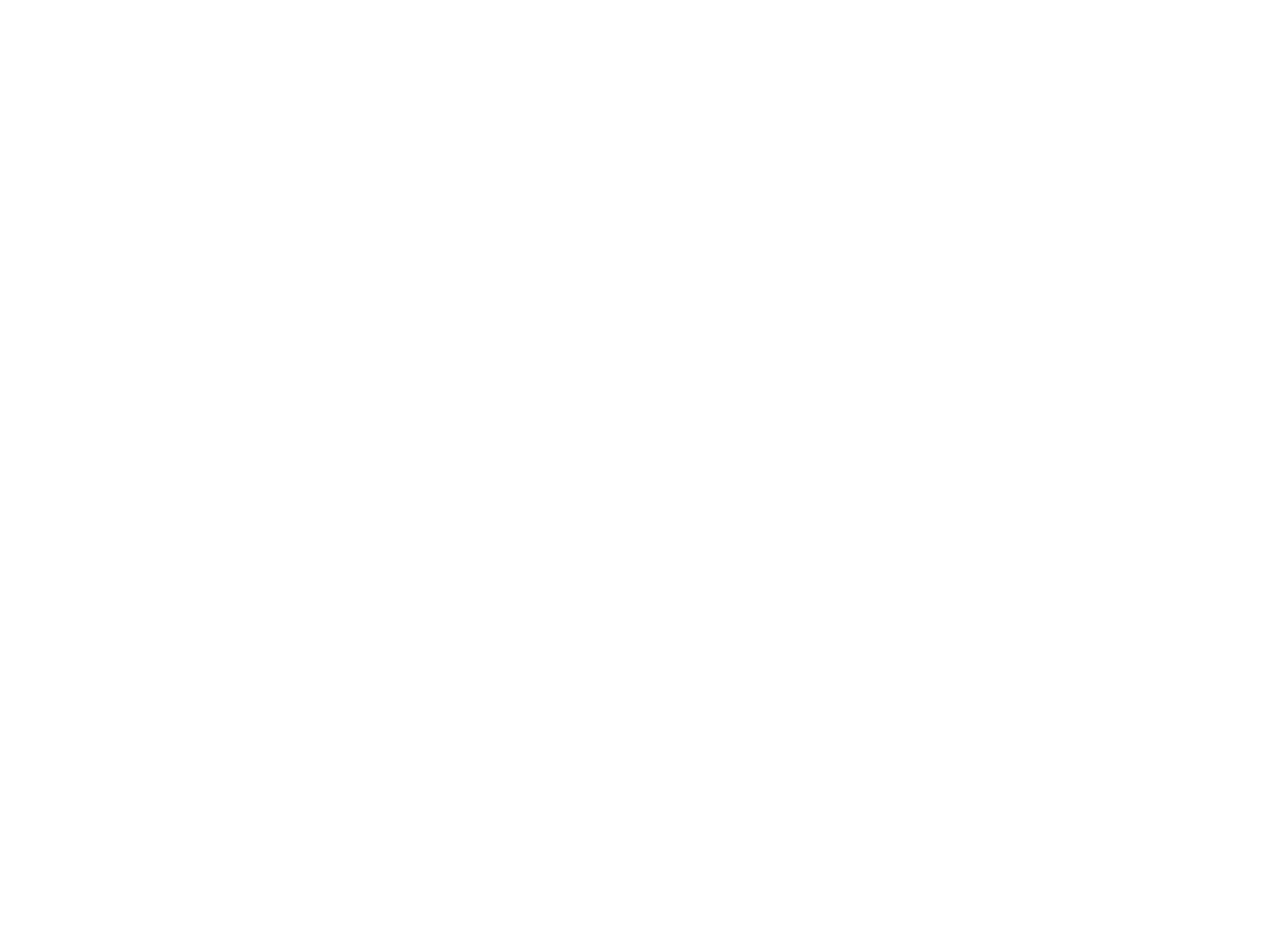
Decoding surgeon activity from surgical videos
In our most recent research, published in Nature Biomedical Engineering, we decode surgeon activity from surgical videos through the use of artificial intelligence (AI). The use of artificial intelligence implies that the decoding of surgeon activity is objective (read: does not depend on the subjective interpretation of a surgeon), reliable (read: consistently provides the correct decoding), and scalable (read: does not require the presence of a surgeon). These qualities suggest that our AI system, which we refer to as SAIS, should be broadly applicable.
Decoding the steps of surgery
Can we reliably distinguish between surgical steps?
We trained SAIS to distinguish between three surgical steps: needle handling, needle driving, and needle withdrawal. These reflect periods of time during which a surgeon first handles a needle to drive it through some tissue before withdrawing it as part of the suturing process. Specifically, to probe SAIS' ability to generalize (read: perform well) to real-world data from different settings, we trained it on data exclusively from the University of Southern California (USC) and deployed it on previously-unseen videos from USC and from completely different hospitals: St. Antonius Hospital, Germany (SAH) and Houston Methodist Hospital, USA (HMH).
We show that SAIS generalizes to unseen surgical videos and those from unseen surgeons at different hospitals. While the former is evident by the strong performance (AUC > 0.90) achieved when SAIS was deployed on USC data, the latter is supported by the strong performance (AUC > 0.85) when SAIS was deployed on data from SAH and HMH.
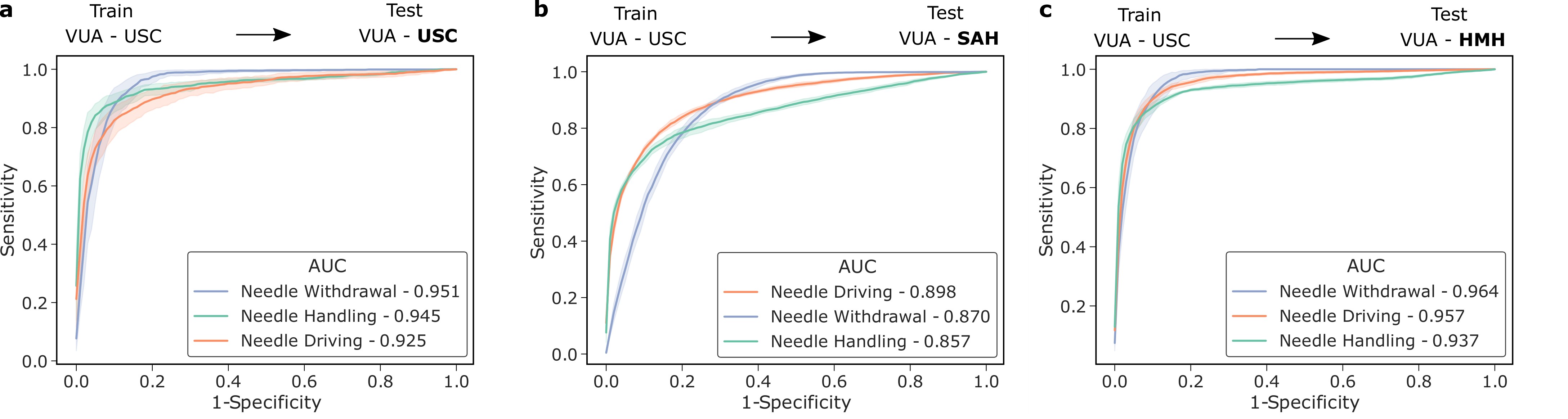
Decoding the gestures of surgery
Can we reliably distinguish between surgical gestures?
We also trained SAIS to distinguish between distinct gestures performed by surgeons. These reflect the decisions made by a surgeon about how to execute a particular surgical step. To emphasize again, SAIS was trained on data exclusively from USC and deployed on unseen videos of the same surgery (RARP) from USC and SAH. In this setting, we also deployed SAIS on videos from an entirely different surgical procedure, known as a robot-assisted partial nephrectomy (RAPN), in which a portion of a patient's kidney is removed to treat cancer.
We show that SAIS generalizes to unseen videos, surgeons, hospitals, and surgical procedures. This is evident by its strong performance (see below) in all of these settings.
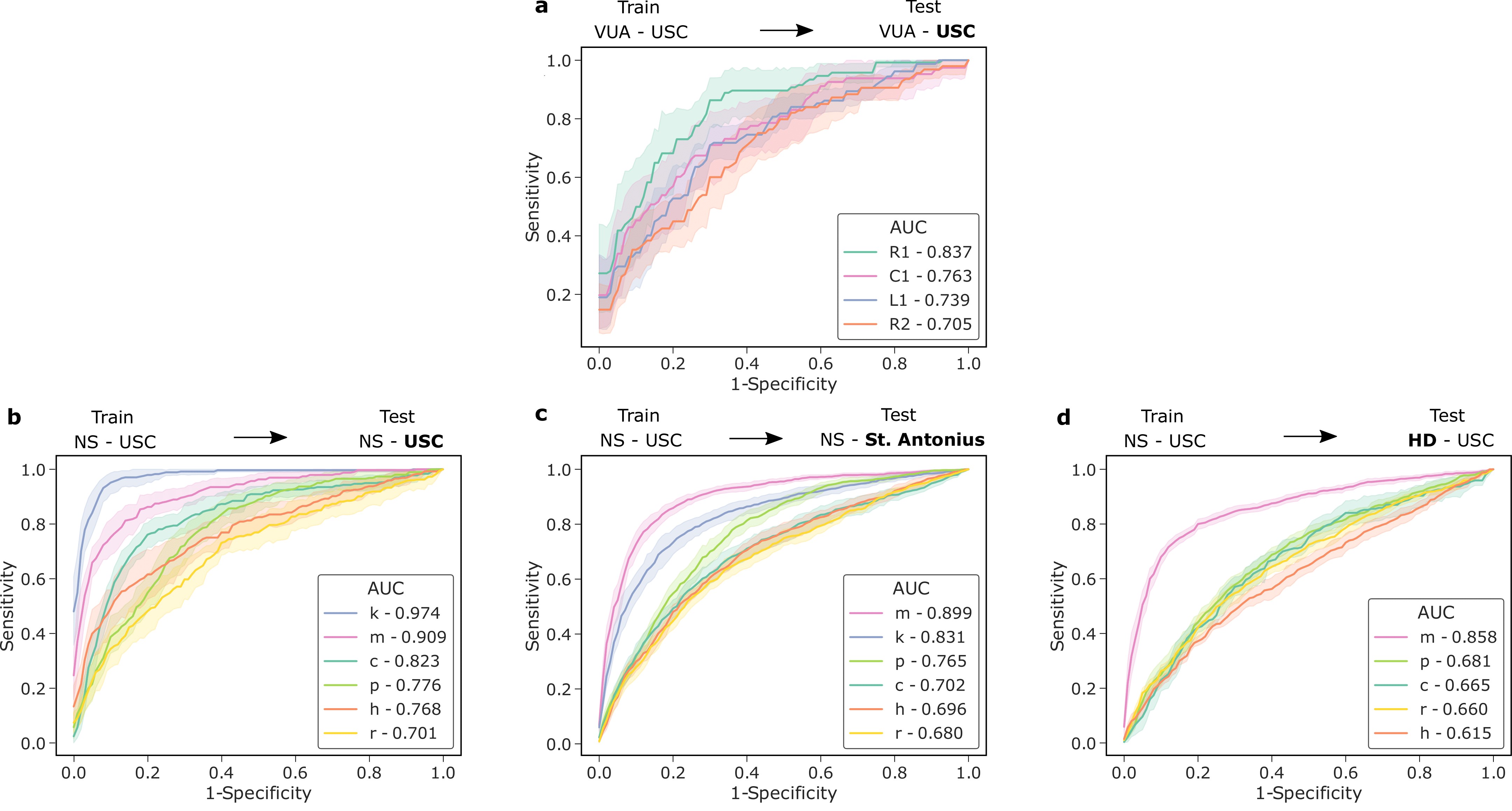
Decoding the skill-level of surgical activity
Can we reliably distinguish between low and high skill activity?
We then trained SAIS to distinguish between low and high skill activity, for two distinct surgical activities: needle handling and needle driving. For needle handling, a low-skill assessment is based on the number of times a surgeon had to reposition their grasp of the needle (more repositions = lower quality). For needle driving, a low-skill assessment is based on the smoothness with which it was performed (less smooth = lower quality).
We show that SAIS generalizes to unseen videos, surgeons, and hospitals. This is evident by its strong performance (AUC > 0.80) across the skill assessment tasks and hospitals. SAIS also naturally lends itself to explainable findings. Below, we illustrate the relative importance of individual frames in a surgical video, as identified by SAIS, and show that the frames with the highest level of importance do indeed align with the ground-truth low-skill assessment of the surgical activity.
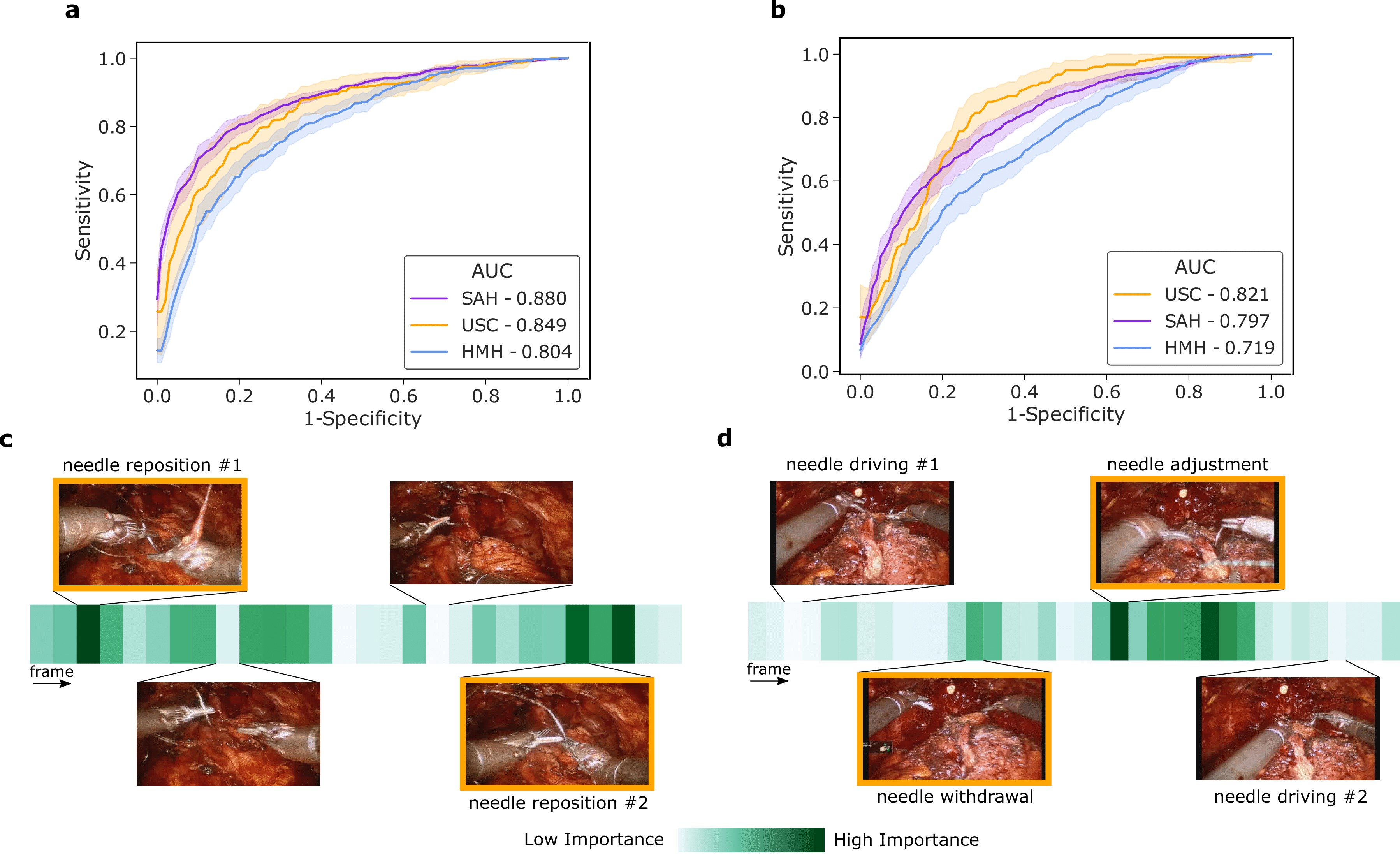
Combining the elements of surgery
How do we practically leverage the findings of this study?
Thus far, we have demonstrated SAIS' ability to independently decode multiple elements of surgical activity (steps, gestures, and skill-levels). Considering these elements in unison, however, suggests that SAIS can provide a surgeon with performance feedback of the following form:
When completing stitch number 3 of the suturing step, your needle handling (what - sub-phase) was executed poorly (how - skill). This is likely due to your activity in the first and final quarters of the needle handling sub-phase (why - explanation).
Such granular and temporally-localized feedback allows a surgeon to better focus on the element of surgery that requires improvement. As such, a surgeon can now better identify, and learn to avoid, problematic intra-operative surgical behaviour in the future.
Outlining the translational impact
What are the implications of our study?
The ability of SAIS to generalize to videos across a wide range of settings (e.g., surgeons, hospitals, and surgical procedures) is likely to instill surgeons with greater confidence in its performance. This opens the door for the large-scale decoding of the millions of surgical videos recorded on an annual basis. Moreover, SAIS' ability to provide explainable findings, by highlighting relevant video frames, has a threefold benefit. First, explainability can improve the trustworthiness of an AI system, a critical pre-requisite for clinical adoption. Second, it allows for the provision of more targeted feedback to surgeons about their performance, thereby allowing them to modulate their behaviour to improve patient outcomes. Third, explainability can improve the transparency of an AI system, and thus contributes to the ethical deployment of AI systems in a clinical setting.
Moving forward
Where do we go from here?
Beyond demonstrating the reliability of SAIS on data from distinct settings, it is critical that we also explore the ethical implications of its deployment. To that end, in a concurrent study published in npj Digital Medicine, we explore the fairness of SAIS' skill assessments and whether it disadvantages one surgeon subcohort over another. One way to leverage SAIS is for the provision of surgeon feedback, and we see its explanations playing a critical role in that process. As such, in a concurrent study published in Communications Medicine, we systematically investigate the quality and fairness of SAIS' explanations.
Acknowledgements
We would like to thank Wadih El Safi for lending us his voice.